The Body Electric: Biomolecules for Batteries
An electrified economy will need better tools for controlling electrons, but we can use ML to borrow some tricks from biology.
Now you can't hold it
But you know it’s there
Here there and everywhere…It’s Electric!
-Marcia Griffiths, Electric Boogie
Electricity generation is responsible for 25% of US emissions [1], and demand is expected to double by 2050 due to electrification of vehicles, heating, and industry [2]. This is one climate problem we know how to solve, however: replace fossil fuels with renewables as quickly as possible. So far, it’s a success story. In 2022, 23% of US electricity came from renewables [3], and more than half of new capacity came from solar photovoltaics (PV) and wind [4]. The scale of the challenge is still huge–to reach net-zero emissions by 2050, we need to double the rate of renewable additions in three years [2]–but in a world with lots of gloomy climate news, the explosive growth of renewable electricity is a cause for hope.
Renewables will allow us to change the foundation of our economy from heat to electrons. Today, we burn fossil fuels and make chemicals and materials at high heat/pressure, releasing CO2 and wasting energy. In an electron-economy, the production of power, chemicals, and materials takes place at room temperature using electrochemical or electromechanical processes. However, realizing this vision will require us to get really good at managing electrons.
For example, solar PV and wind force us to manage variability in electron supply, due to daily and seasonal cycles which we can’t control and weather events which we can’t predict. Once grid capacity is ~80% renewables, grid operators have few tools to meet demand when solar and wind dip [5]. A system whose annual capacity matched annual demand would have 80+ days where instantaneous supply could not meet instantaneous demand [5]. In a system of 100% renewables, meeting demand at all hours requires massive overdeployment of 5-8 times peak capacity, unless we ration electricity [6]. Because the marginal cost of electricity skyrockets in those doldrums, getting from 80% to 100% renewables is extremely challenging.
Top-down electricity modelers often advocate meeting this demand with “firm” low-carbon assets like nuclear energy or natural gas with carbon capture [2,6]. However, the widespread use of nuclear energy is hampered by risks (real and perceived) of accidents, waste, and proliferation. Continued reliance on natural gas maintains the political and economic power of fossil fuel companies, and they might not use their reserves judiciously once they’ve deployed the capital to develop a project. Most pragmatically, both options have much higher capital costs than renewables, and they’re more expensive most hours of the year.
The key to a grid with 100% renewables is Long-Duration Energy Storage (LDES), systems which can discharge at full power for 10+ hours. Grid operators already use Lithium-ion (Li-ion) batteries to shift load by a few hours [7]. Unfortunately, Li-ion batteries are uneconomical for LDES because they have a high capex for storage capacity (>$200/kWh) and can typically only discharge for 4-6 hours. In contrast, recent TEAs suggest that LDES must cost less than $20/kWh with 100-hr storage to have a true impact [8].
One promising technology for LDES is the flow battery. We’ll dig into the tech behind flow batteries below, but any battery needs charge-carrying molecules called electrolytes. Most flow batteries today use electrolytes made with vanadium, a rare, expensive element mostly mined in Russia and China. It also suffers from low energy density and electrode degradation.
That’s where biology comes in: cells have evolved to manipulate electrons with nanoscale precision, repeatedly for decades. For example, every animal cell has >1000 mitochondria, which use high energy electrons to make ATP, the chemical fuel for life. Figure 1 shows the mitochondrial “electron transport chain,” where electrons flow between biomolecular machines to drive ATP synthesis. The exquisite spatial and temporal control of electrons in mitochondria arguably surpasses any engineered system, and it’s happening in every cell all the time.
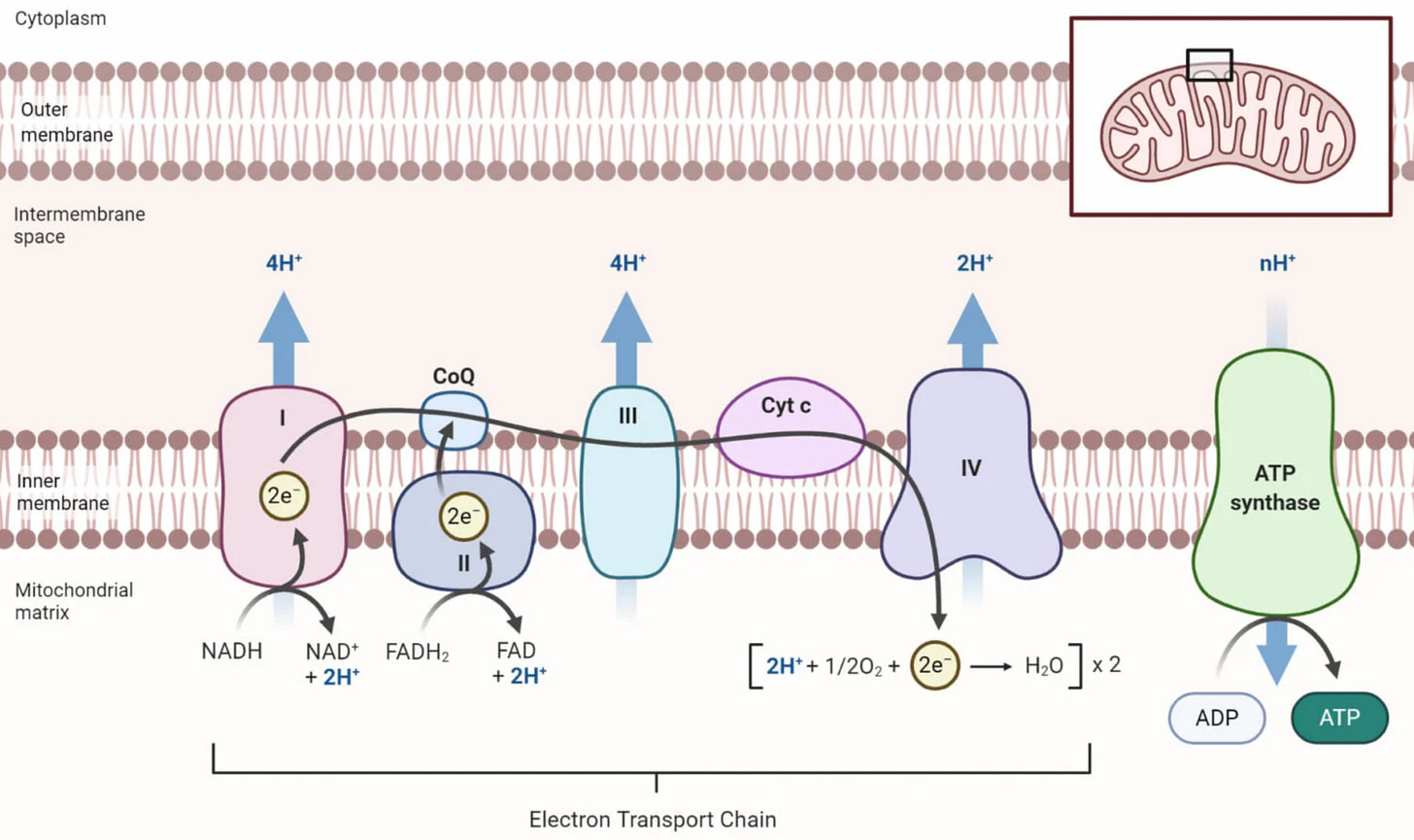
Can we harness this biological control of electrons, for example by using biomolecules as electrolytes? We think biotech can help us control electrons to build a renewable electron-economy, and we’re going to explore applications in LDES and beyond.
The tech
To understand how biomolecules can help us control electrons for energy storage, let’s take a look at how batteries work. The idea behind a battery is the same as a water tower: when we have extra energy, we pump water uphill into a state with higher gravitational potential energy. When we need energy, we release the water and use it to spin a turbine.1 A battery operates using the same principle, only instead of pumping water up a gravitational potential, we pump electrons up an electrical potential. (Confession: we defined “positive” and “negative” before we discovered electrons, so the negatively-charged electrons like to flow uphill to higher potential. Blame Benjamin Franklin.)
It’s a simple physical picture, but building a practical device requires some chemistry. Different atoms and molecules have different “redox potentials,” and electrons flow from materials with low redox potential to materials with higher potentials, like water flowing downhill. The simplest “battery” imaginable is the Galvanic Cell, pictured in Fig. 2. Here, zinc has a lower redox potential than copper, so electrons will flow from the zinc to copper if we connect them with a wire. Then we can place a “load,” such as a lightbulb or a laptop, on the wire and use the flowing electrons for energy!
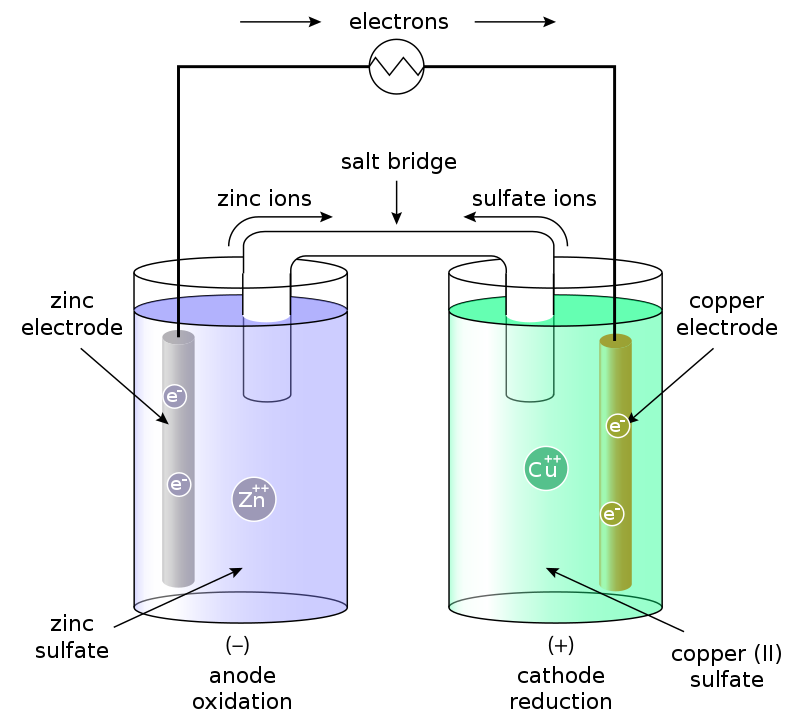
Is that it? Just put together two materials with different redox potentials and we have electricity? Clearly not, or else the whole world would be a battery. This is where batteries are different from water towers. Electrons have charge, so pumping them creates a charge imbalance that quickly cancels out the redox potentials. The solution shared by all batteries is ion exchange, used to maintain an electrically-neutral system as electrons flow from one cell to another.
For example, the Galvanic Cell uses a salt bridge to exchange sulfate ions. When the zinc loses its electrons, it goes from solid zinc, Zn(s), to a positive zinc ion, Zn2+, which dissolves in water. Conversely, as the dissolved copper ions gain electrons, they become neutral solids and ditch their sulfate anions. The salt bridge allows the sulfate anions to move between solutions to return the system to neutral charge, “completing the circuit.” This reaction is expressed compactly in the following chemical equations (N.B. the aqueous sulfate ions, which jump across the salt bridge to balance charge):
And voila, we have a battery! The electron-donating material (zinc) is known as the “anode” and the electron-receiving material (copper) as the “cathode” (My AP Chem teacher taught us this as FAT CAT: From Anode To CAThode). The sulfate solution is an “electrolyte” which allows ions to flow between them.
All batteries are variations on this basic idea. Lithium-ion batteries use solid Lithium compounds as the cathode and anode (e.g. Li-graphite cathodes and Li-Cobalt Oxide anodes) and Li+ itself as the exchanged ion. Li-ion batteries have many advantages which have made them the chemistry of choice for electronics and EVs. They are energy dense, and the high reversibility of the reactions as well as Lithium’s high mobility make it possible to recharge them many times.
Although Li-ion batteries are great for phones and cars, they scale poorly for the engineering needs of LDES. Because the ion exchange is Li jumping between solids, you can’t increase energy capacity (i.e. add more solids) without increasing the interfacial surface area. As a result, capacity and power are linked, making it uneconomical to build battery packs which last more than 4-6 hours. Li-ion batteries also suffer from global competition for scarce resources and safety issues, making them unfit for LDES.
A promising alternative is the Redox Flow Battery. Flow batteries forgo solid ion scaffolds, instead storing ions in separate tanks of liquid electrolytes. The electrolytes flow into a cell with electrodes and a semi-permeable membrane for ion transfer. Figure 3 shows a popular configuration using vanadium compounds for both electrolytes.
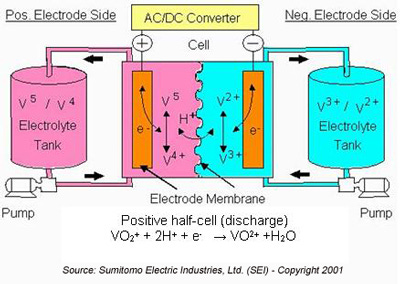
This setup has some major advantages for LDES. The storage capacity is limited by external tanks rather than the amount of material in the cell, so you can add new capacity modularly. Moreover, discharge rates can be controlled by the flow rate of the electrolytes. This provides flexibility to the changing demands of the grid.
Vanadium ions have been the most successful anolyte and catholyte (liquid anodes and cathodes). However, vanadium is limited by its scarcity, geographical distribution, high price, and low energy/current density. So the hunt for alternative options is on! Organic molecules, inspired by the electrochemistry of biology, are a promising option for scalable and sustainable flow batteries.
Quinones are a class of organic molecules that are well-suited to performing redox reactions. They can exist in 9 redox states, with the ability to accept two electrons and two protons. Figure 4 shows an example quinone redox cycle. Additionally, they are stable and have high chemical diversity. These properties have allowed quinones to play a major role in aerobic respiration, the energy production system of most multicellular organisms [9].
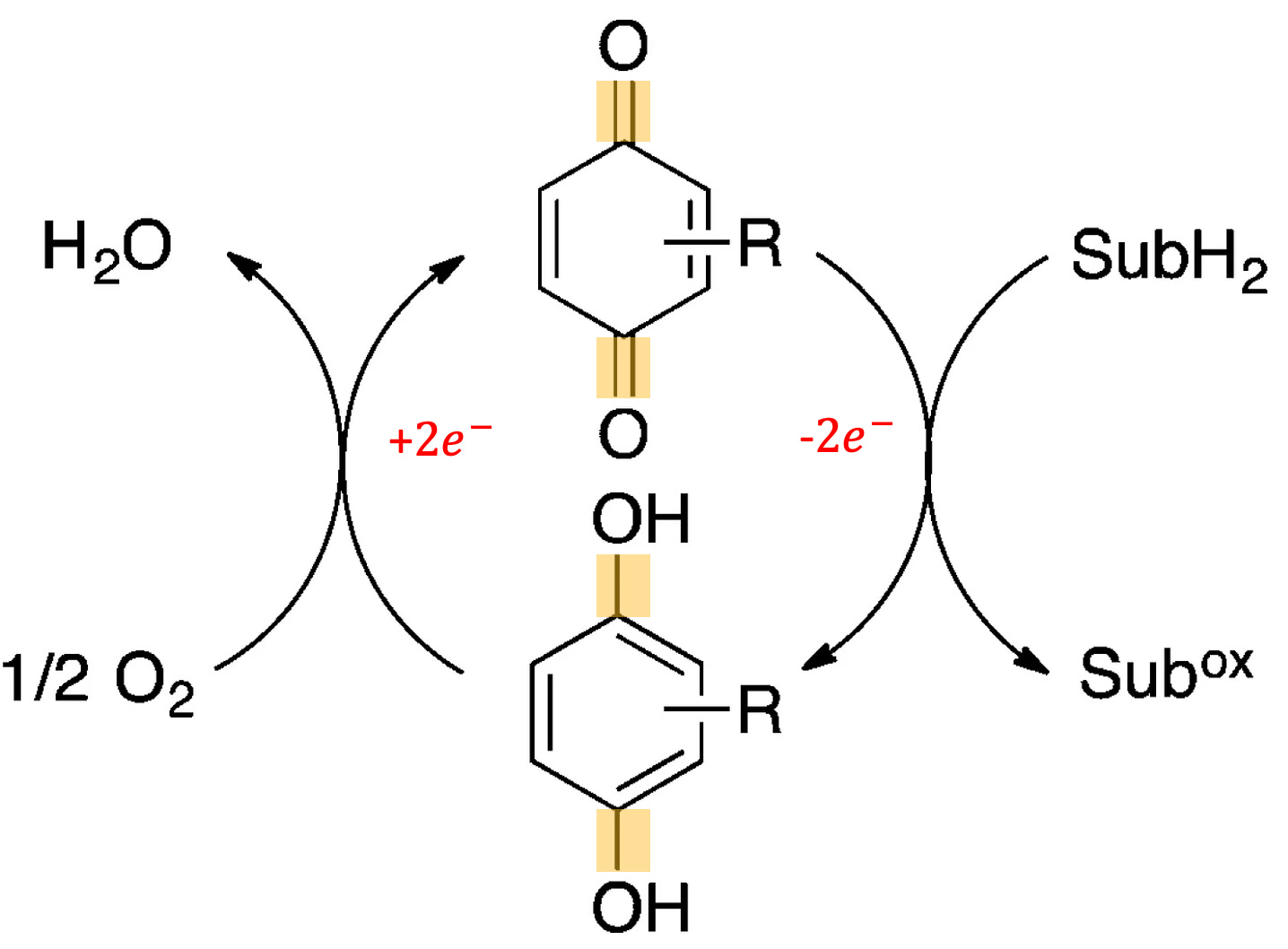
Several academic groups have developed prototype quinone batteries [11-13]. However, these efforts are limited by the molecules nature gave us, and evolution has not selected for use in flow batteries. For example, we need molecules that allow us to achieve high energy density, which depends on the number of electrons carried as well as the solubility and the redox potential. Can we use ML to design organic molecules to our own specifications?
A Bio + ML opportunity
Machine learning techniques have successfully been used in biology to develop novel molecules for everything from antibiotics [14, 15] to bioweapons [16]. The challenge is to computationally predict a molecule’s properties from its structure (or to generate structures with desired properties). Neural networks are “universal function approximators,” so they should be up to the task if we can provide the right data and the right network setup. For example, graph convolution is a promising neural network architecture for modeling chemical structures like quinones. Several organic reaction chemistries have been successfully modeled with neural networks [17]. The challenge for flow battery molecule design is balancing different engineering requirements. In addition to energy density, molecules need to have:
Favorable reaction kinetics between anolyte and electrolyte
The correct permeability (or lack thereof) through the membrane
High solubility and low viscosity in the chosen solvent
Long term stability for safety and life span
…and other engineering properties.
While complex, multi-task models could be used to simultaneously design anolyte and catholyte together across multiple properties, the computational and experimental challenges are non-trivial. Complex neural network models require lots of data to train, on the order of tens of thousands of data points to effectively use “overparameterized” architectures. A large library of potential quinones would need to be synthesized for experimentation. While large libraries of chemical molecules exist, these tend to be focused on potential bioactive pharmaceuticals. Then collecting high quality experimental data for lots of screens would require the construction of a high-throughput test system that can accurately measure multiple variables relevant for flow batteries.
Computationally, representing chemical structures is still an open challenge. Structures have been represented in one dimension as a string of elements with perhaps some relevant properties, in two dimensions as a connected graph of atoms, and in three dimensions as distributions of spatial positions of the atoms. Higher dimensional representations capture more information about the molecule, but require greater memory, compute power, and model complexity. Another open challenge is generating novel chemical structures that are valid, given the complexity of chemical space.
Nevertheless, the integration of machine learning into the design process of redox flow battery materials could accelerate discovery of molecular compounds that boost performance and lower cost. AI systems may also be helpful in the battery control decision making and fluid dynamic design. Generalizing the techniques from machine learning for drug discovery to redox chemistry is an achievable goal with a clear impact for LDES and other electrochemical processes.
Market analysis
The future market size for LDES depends on how the entire power system evolves through an unprecedented energy transition. We don’t know what technologies will be available at what cost, nor how markets will vary across regions with different geographies and policies. One way to handle so much uncertainty is to calculate the “lowest cost” system based on constraints like estimated demand, local weather patterns, technological learning curves, and the structure of electricity markets. Such models will only be as good as their inputs, and real actors competing on the ground don’t always arrive at globally optimal solutions, so you should take these models with a grain of salt.
Given all those caveats, however, the opportunity appears to be large. Today, the global energy and power capacity of LDES are 65GWh and 5GW, respectively. McKinsey & Co. used a proprietary power model to predict that by 2040, those numbers will balloon to ~100,000GWh of energy and 2,000GW of power, making up a $1.5-3 trillion global market [18]. The Net-Zero America project doesn’t consider LDES, but it estimates that the US will require 500-1000GW of “low-C firm capacity” in 2050. That means there could be a demand for LDES equivalent to today’s total US coal, gas, and nuclear output. No matter how you run the numbers, LDES presents a huge opportunity for climate and business.
For organic redox flow batteries to reach that scale, however, they need to offer lower cost or higher value than their competitors. In the design space for LDES systems, the three most important parameters for market competitiveness are [8]:
Power capex (USD/kW), where power is energy/time,
Capacity capex (USD/kWh), where capacity is how much energy can be stored, and
Round-trip efficiency, the fraction of charging energy available upon discharge.
The duration of full discharge at full power is also important. In [8], they find that capacity capex must fall below $20/kWh to compete with low-C firm generation, preferably with a discharge time of 100+ hours (4+ days). At $1/kWh, LDES can displace firm generation entirely. In comparison, Li-ion batteries can cost hundreds/kWh, and even optimistic predictions for future cost reductions don’t bring them below $100/kWh.
Are these targets achievable for LDES? McKinsey & Co. have analyzed the average costs across a variety of LDES technologies (everything from flow batteries to compressed air to piles of molten salt) and applied learning rates based on historical data from solar PV and wind, as well as from earlier iterations of LDES technologies [18]. Figure 5 shows that capacity costs could comfortably fall below $40/kWh, with costs below $10/kWh plausible for longer-duration technologies. Thus the targets from [8], though ambitious, are in line with technological learning demonstrated by other energy technologies.
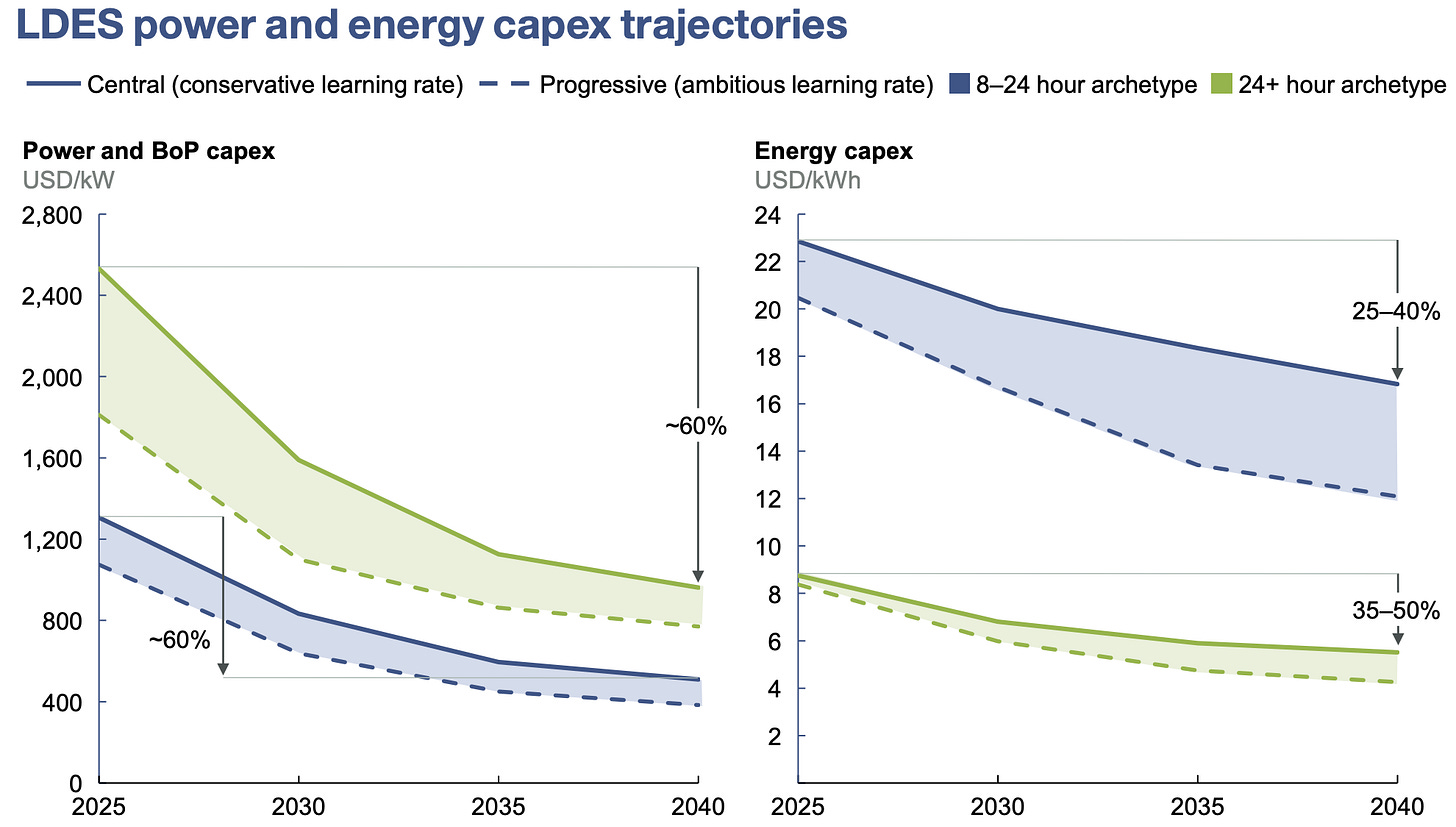
For redox flow batteries, the main cost drivers are electrolytes and the power stack (electrodes+membranes), which together make up 60–70% of costs [19]. ML-optimized bio-electrolytes can make progress on both fronts. Vanadium is relatively expensive ($24/kg), compared to $5/kg expected in the long term for bio-electrolytes. Stack costs are related to current density: the higher the density, the smaller the membrane. Organic molecules can be optimized to increase current density by tuning solubility, transport, and electron-transfer kinetics. On top of all this, almost 90% of vanadium is produced by China and Russia, creating geopolitical risk in the supply chain [20], whereas bio-electrolytes can be produced with abundant elements found anywhere.
Flow batteries using organic electrolytes are an emerging technology, but there are a few companies already trying to deploy them. In 2023, the German company CMBlu is building 1MWh pilot projects at power plants in the US and Germany. [21, 22]. CMBlu is close-lipped about their technology, but based on interviews and patents they appear to have a process for converting lignin–a byproduct of paper pulping–into a redox-active molecule. Another German company, CERQ (formerly Jena Batteries) is developing an amine-based battery with a capacity of 400kWh in collaboration with BASF [23]. The French KemiWatt is also a competitor, although details on their technology and R&D stage are scarce. Other early players include Rivus (Sweden), which hopes to test its electrolyte in a small battery in 2023, and Quino (US), a quinone-based company which recently raised a $4.5 seed round. In spite of this technology’s huge potential, the field is still wide open. We don’t have much visibility into the electrolyte design process for these companies. However, based on the public literature and what little info is available, it seems likely that the electrolytes are natural or with small, expert-based modifications. In contrast, a ML approach to molecular design could open up previously unimagined opportunities.
For organic flow batteries to succeed, however, they need to compete in the broader LDES marketplace, not just against other flow batteries. Form Energy is developing an iron-air battery for 100+ hour storage. Form has a substantial head start, with a $450 million Series E round in 2022 [24], and two 1MWh pilots set to come online in 2025 [25]. On the other hand, the promise of electron control via bespoke biomolecules goes far beyond LDES.
So many processes in the chemical and industrial sectors use high heat and pressure, making them inefficient and difficult to electrify. Decarbonization will require a transition towards electrochemistry, where careful control of electrons allows us to make materials and chemicals at room temperature with clean electricity. Biomolecules have evolved to control electrons, and ML can help us leverage this rich chemical space to build the clean electron-economy.
Conclusion
The transition to renewable electricity promises a smorgasbord of clean electrons, but reaching net-zero emissions will require new tools to handle them. One particularly important application is long-duration energy storage, which we’ll need to power the grid with 100% renewables. Organic flow batteries provide scalable LDES, and using ML and modern synthetic biology will allow us to optimize them for low cost with cheap, abundant, locally-sourced elements. More broadly, engineering biomolecules to manipulate electrons will allow us to decarbonize power, chemicals, and manufacturing and build an economy that utilizes electrons as effectively as our bodies do.
Next time: Tune in to learn how microbes can help mitigate methane emissions from landfills!
References
[1] US Environmental Protection Agency. Sources of Greenhouse Gas Emissions. 2023. url: https:/ /www.epa.gov/ghgemissions/sources-greenhouse-gas-emissions.
[2] Eric Larson et al. “Net-Zero America: Potential Pathways, Infrastructure, and Impacts, Final report”. Princeton University (2021).
[3] Bloomberg NEF. Sustainable Energy in America 2023 Factbook. 2023. url: https://bcse.org/market-trends/.
[4] US Energy Information Administration. Solar power will account for nearly half of new U.S. electric generating capacity in 2022. 2022. url: https://www.eia.gov/todayinenergy/detail.php?id=50818#.
[5] Dan Tong et al. “Geophysical constraints on the reliability of solar and wind power worldwide”. In: Nature communications 12.1 (2021), p. 6146.
[6] Nestor A Sepulveda et al. “The role of firm low-carbon electricity resources in deep decarbonization of power generation”. In: Joule 2.11 (2018), pp. 2403–2420.
[7] ARPA-E. Why Long-Duration Energy Storage Matters. 2020. url: https:/ /arpa-e.energy.gov/news-and-media/blog-posts/why-long-duration-energy-storage-matters.
[8] Nestor A Sepulveda et al. “The design space for long-duration energy storage in decarbonized power systems”. In: Nature Energy 6.5 (2021), pp. 506–516.
[9] Jennifer Madeo, Adeel Zubair, and Frieri Marianne. “A review on the role of quinones in renal disorders”. In: Springerplus 2 (2013), pp. 1–8.
[10] Mioy T Huynh et al. “Quinone 1 e–and 2 e–/2 H+ reduction potentials: Identification and analysis of deviations from systematic scaling relationships”. In: Journal of the American Chemical Society 138.49 (2016), pp. 15903–15910.
[11] Giyun Kwon et al. “Bio-inspired molecular redesign of a multi-redox catholyte for high-energy non-aqueous organic redox flow batteries”. In: Chem 5.10 (2019), pp. 2642-2656.
[12] Mikhail Vagin et al. “Ion-Selective Electrocatalysis on Conducting Polymer Electrodes: Improving the Performance of Redox Flow Batteries”. In: Advanced Functional Materials 30.52 (2020), p. 2007009.
[13] Bo Yang et al. “A durable, inexpensive and scalable redox flow battery based on iron sulfate and anthraquinone disulfonic acid”. In: Journal of The Electrochemical Society 167.6 (2020), p. 060520.
[14] Gary Liu et al. “Deep learning-guided discovery of an antibiotic targeting Acinetobacter baumannii”. In: Nature Chemical Biology (2023), pp. 1–9.
[15] Cesar de la Fuente-Nunez. “Antibiotic discovery with machine learning”. In: Nature Biotechnology 40.6 (2022), pp. 833–834.
[16] Fabio Urbina et al. “Dual use of artificial-intelligence-powered drug discovery”. In: Nature Machine Intelligence 4.3 (2022), pp. 189–191.
[17] Zhengkai Tu, Thijs Stuyver, and Connor W Coley. “Predictive chemistry: machine learning for reaction deployment, reaction development, and re action discovery”. In: Chemical Science (2023).
[18] LDES Council and McKinsey Co. Net-zero power: Long-duration energy storage for a renewable grid. 2021. url: https://www.mckinsey.com/capabilities/sustainability/our-insights/net-zero-power-long-duration-energy-storage-for-a-renewable-grid.
[19] L Tang et al. “Capital cost evaluation of conventional and emerging redox flow batteries for grid storage applications”. In: Electrochimica Acta 437 (2023), p. 141460.
[20] USGS. Mineral Commodity Summaries: Vanadium. 2023. url: https://pubs.usgs.gov/periodicals/mcs2023/mcs2023-vanadium.pdf.
[21] CMBlu Energy. CMBlu Energy Continues U.S. Expansion With Long- Duration Energy Storage Pilot Project in Wisconsin. 2023. url: https://www.cmblu.com/en/press-and-media/cmblu-us-expansion/.
[22] CMBlu Energy. Uniper and CMBlu Energy intensify collaboration for the transformation of the Staudinger power plant site in Hesse, Germany. 2023. url: https://www.cmblu.com/en/press-and-media/uniblu-uniper-cmblu-pilotprojekt-transformation/.
[23] BASF. Jena Batteries and BASF cooperate in the development of innovative power storage technology. 2020. url: https://www.basf.com/global/en/media/news-releases/2020/02/p-20-125.html.
[24] Form Energy. Form Energy Announces $450M Series E Financing. 2022. url: https://formenergy.com/form-energy-announces-450m-series-e-financing/.
[25] Form Energy. Form Energy Partners with Xcel Energy on Two Multi-day Energy Storage Projects. 2023. url: https://formenergy.com/form-energy-partners-with-xcel-energy-on-two-multi-day-energy-storage-projects/
Stored pumped hydro is the most common form of LDES in the world, but it’s geographically constrained.